From the Journal: Applied Physics Reviews
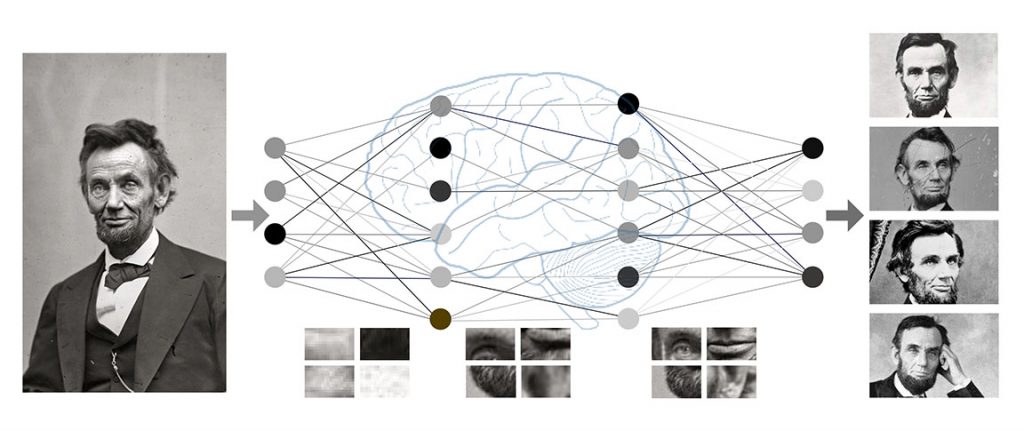
WASHINGTON, D.C., January 15, 2020 — Since the invention of the transistor in 1947, computing development has seen a consistent doubling of the number of transistors that can fit on a chip. But that trend, known as Moore’s Law, may reach its limit as components of submolecular size encounter problems with thermal noise, making further scaling impossible.
In their paper published this week in Applied Physics Reviews, from AIP Publishing, authors Jack Kendall, of Rain Neuromorphics, and Suhas Kumar, of Hewlett Packard Labs, present a thorough examination of the computing landscape, focusing on the operational functions needed to advance brain-inspired neuromorphic computing. Their proposed pathway includes hybrid architectures composed of digital architectures, alongside a resurgence of analog architectures, made possible by memristors, which are resistors with memory that can process information directly where it is stored.
“The future of computing will not be about cramming more components on a chip but in rethinking processor architecture from the ground up to emulate how a brain efficiently processes information,” Kumar said.
“Solutions have started to emerge which replicate the natural processing system of a brain, but both the research and market spaces are wide open,” Kendall added.
Computers need to be reinvented. As the authors point out, “Today’s state-of-the-art computers process roughly as many instructions per second as an insect brain,” and they lack the ability to effectively scale. By contrast, the human brain is about a million times larger in scale, and it can perform computations of greater complexity due to characteristics like plasticity and sparsity.
Reinventing computing to better emulate the neural architectures in the brain is the key to solving dynamical nonlinear problems, and the authors predict neuromorphic computing will be widespread as early as the middle of this decade.
The advancement of computing primitives, such as nonlinearity, causality and sparsity, in new architectures, such as deep neural networks, will bring a new wave of computing that can handle very difficult constrained optimization problems like weather forecasting and gene sequencing. The authors offer an overview of materials, devices, architectures and instrumentation that must advance in order for neuromorphic computing to mature. They issue a call to action to discover new functional materials to develop new computing devices.
Their paper is both a guidebook for newcomers to the field to determine which new directions to pursue, as well as inspiration for those looking for new solutions to the fundamental limits of aging computing paradigms.
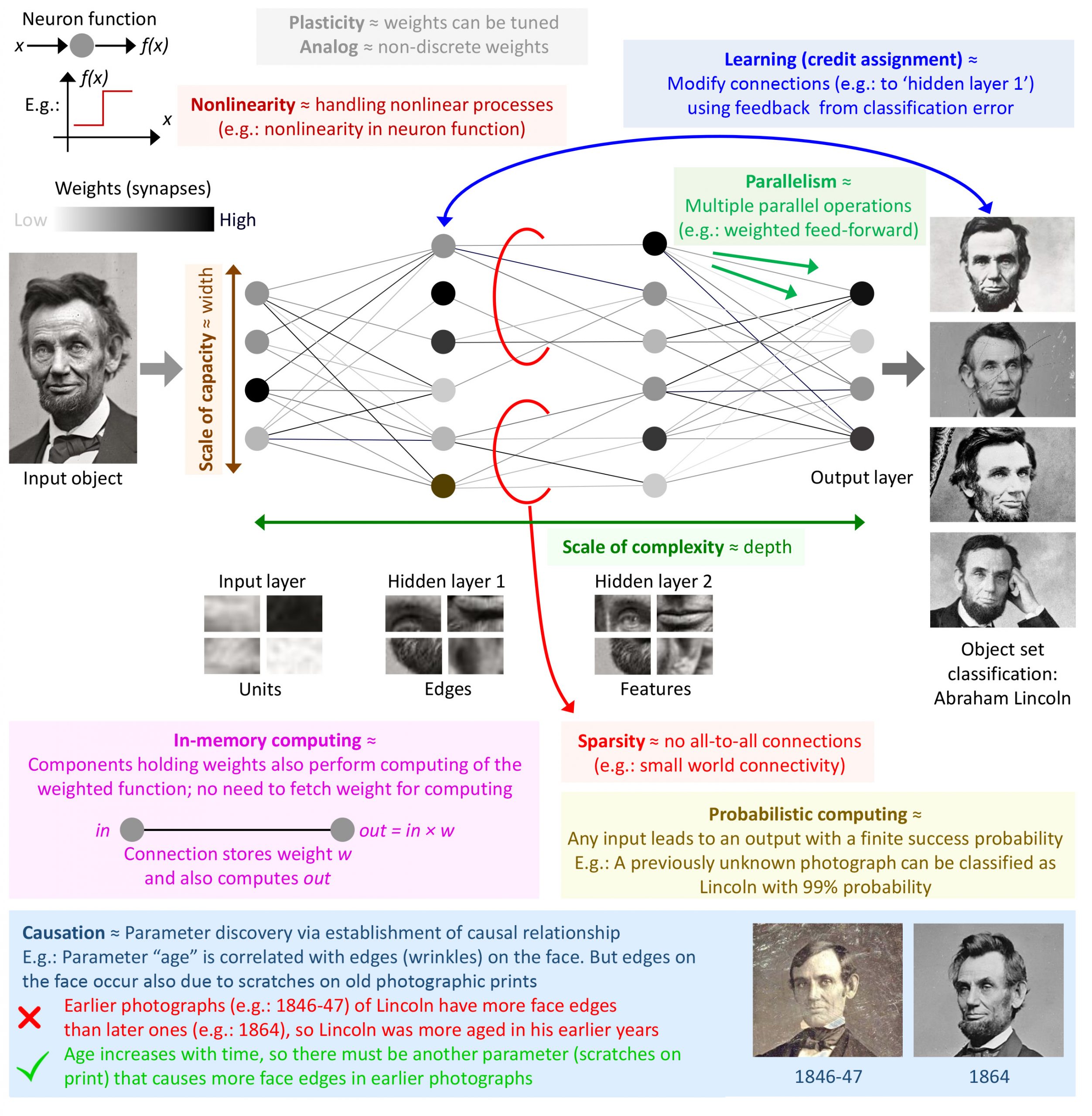
###
For more information:
Larry Frum
media@aip.org
301-209-3090
Article Title
The building blocks of a brain-inspired computer
Authors
Jack D. Kendall and Suhas Kumar
Author Affiliations
Rain Neuromorphics, Hewlett Packard Labs