Integrating Data Science and Computational Materials Science
Submission Deadline: June 30, 2025Contribute to this Special Topic
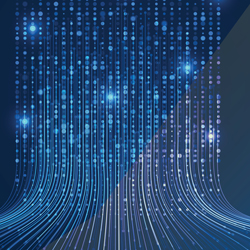
Topics covered include, but are not limited to:
- New techniques that integrate data science and computational material science including explainable AI methods, graph neural networks, large language models, and reinforcement learning
- Addressing current challenges to integrate computational material science and data science, and proposing new strategies for overcoming them
- Accelerating computational materials science through machine learning to overcome the limitations of traditional computational techniques
- Computational materials design and discovery to guide experimental investigations such as energy storage, sustainable technologies, and aerospace, etc.
- Development of open-access data repositories, software, and techniques that assist computational materials science approaches promoting collaboration and transparency in the community
- Standardization of materials data to ensure the reproducibility of computational models
Guest Editors
Dilpuneet S. Aidhy, Clemson University
Donghwa Lee, Pohang University of Science and Technology
Kamal Choudhary, National Institute of Standards and Technology
Submission Deadline: June 30, 2025Contribute to this Special Topic