From the Journal: Biophysics Reviews
WASHINGTON, September 28, 2021 — Whole-heart ventricular modeling has come a long way in recent years and is currently witnessing the evolution of a variety of computational approaches, especially within the realm of personalized technologies for patient-specific clinical applications.
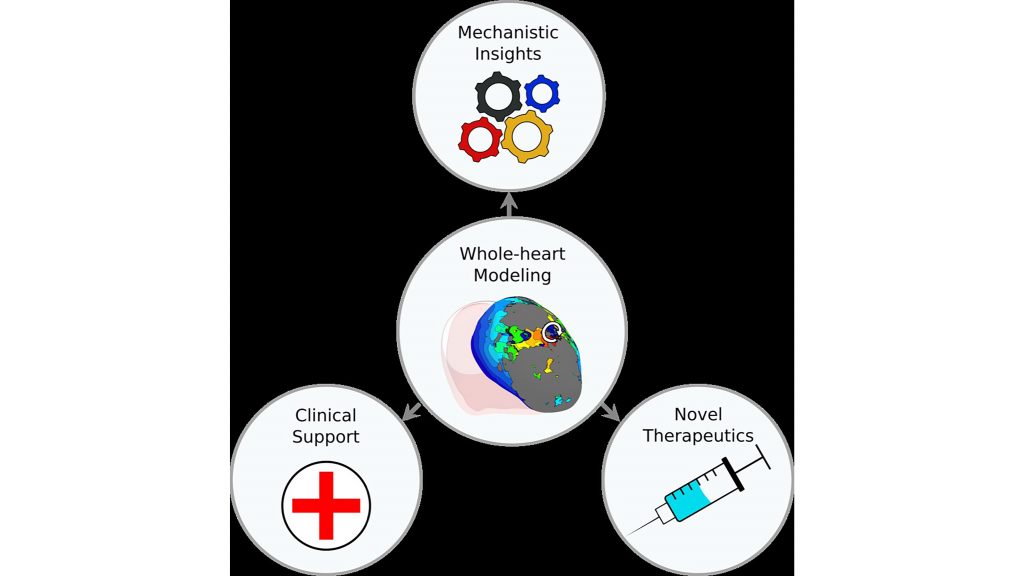
Ventricular arrhythmias, which are abnormal heartbeats, are one of the leading causes of mortality worldwide. To pursue a better mechanistic understanding of ventricular arrhythmias, Johns Hopkins University researchers are turning to whole-heart computational models.
In Biophysics Reviews, from AIP Publishing, the researchers describe the progress using various computational approaches to address the mechanisms of cardiac dysfunction and issues related to the clinical application of computation-driven diagnostic and therapeutic approaches for cardiac disease and arrhythmias.
The heart’s electrical properties can be modeled via fundamental biophysical principles determined through basic science experiments.
Whole-heart computational models are multiscale, which means they factor in both cellular- and organ-level properties. These models include most of the biophysical complexity of an individual patient’s cardiac pathology.
This complex biophysical system “can be represented using a set of mathematical equations,” said Natalia A. Trayanova, a co-author and professor of biomedical engineering and medicine at Johns Hopkins University. “Solving these equations using computer software allows us to run detailed simulations to mimic the heart’s electrical activity.”
Computational models of the heart linking cellular electrophysiology to whole-organ behavior are emerging as promising platforms for in-silico evaluation of novel diagnostic and therapeutic strategies.
“Personalized computational modeling of patient hearts is making strides developing models that incorporate the individual geometry and structure of the heart, as well as other patient-specific information,” Trayanova said.
These patient-specific models can help predict risk of sudden cardiac death or the outcome of a cardiac procedure.
“Patient-specific models are also used for determining the optimal treatment for arrhythmia, both atrial and ventricular, with the latter often based on different biophysical underpinnings,” said Trayanova. “These types of models can enable fast evaluation of medical device settings and patient-selection criteria, as well as the development of novel therapeutic agents.
“Computational modeling can also be combined synergistically with machine learning approaches to better account for the information available within patient health records.”
###
For more information:
Larry Frum
media@aip.org
301-209-3090
Article Title
Authors
Eric Sung, Sevde Etoz, Yingnan Zhang, and Natalia A. Trayanova
Author Affiliations
Johns Hopkins University