From the Journal: Chaos
WASHINGTON, May 19, 2020 — As the infectious virus causing the COVID-19 disease began its devastating spread around the globe, an international team of scientists was alarmed by the lack of uniform approaches by various countries’ epidemiologists to respond to it.
Germany, for example, didn’t institute a full lockdown, unlike France and the U.K., and the decision in the U.S. by New York to go into a lockdown came only after the pandemic had reached an advanced stage. Data modeling to predict the numbers of likely infections varied widely by region, from very large to very small numbers, and revealed a high degree of uncertainty.
Davide Faranda, a scientist at the French National Centre for Scientific Research (CNRS), and colleagues in the U.K., Mexico, Denmark, and Japan decided to explore the origins of these uncertainties. This work is deeply personal to Faranda, whose grandfather died of COVID-19; Faranda has dedicated the work to him.
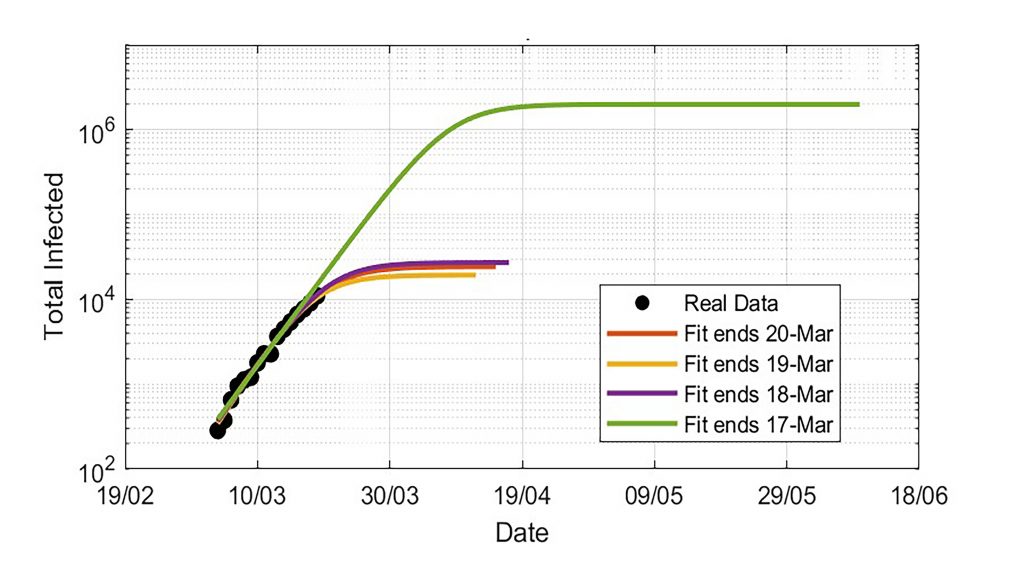
In the journal Chaos, from AIP Publishing, the group describes why modeling and extrapolating the evolution of COVID-19 outbreaks in near real time is an enormous scientific challenge that requires a deep understanding of the nonlinearities underlying the dynamics of epidemics.
Forecasting the behavior of a complex system, such as the evolution of epidemics, requires both a physical model for its evolution and a dataset of infections to initialize the model. To create a model, the team used data provided by Johns Hopkins University’s Center for Systems Science and Engineering, which is available online at https://systems.jhu.edu/research/public-health/ncov/ or https://github.com/CSSEGISandData/COVID-19.
“Our physical model is based on assuming that the total population can be divided into four groups: those who are susceptible to catching the virus, those who have contracted the virus but don’t show any symptoms, those who are infected and, finally, those who recovered or died from the virus,” Faranda said.
To determine how people move from one group to another, it’s necessary to know the infection rate, incubation time and recovery time. Actual infection data can be used to extrapolate the behavior of the epidemic with statistical models.
“Because of the uncertainties in both the parameters involved in the models — infection rate, incubation period and recovery time — and the incompleteness of infections data within different countries, extrapolations could lead to an incredibly large range of uncertain results,” Faranda said. “For example, just assuming an underestimation of the last data in the infection counts of 20% can lead to a change in total infections estimations from few thousands to few millions of individuals.”
The group has also shown that this uncertainty is due to a lack of data quality and also to the intrinsic nature of the dynamics, because it is ultrasensitive to the parameters — especially during the initial growing phase. This means that everyone should be very careful extrapolating key quantities to decide whether to implement lockdown measures when a new wave of the virus begins.
“The total final infection counts as well as the duration of the epidemic are sensitive to the data you put in,” he said.
The team’s model handles uncertainty in a natural way, so they plan to show how modeling of the post-confinement phase can be sensitive to the measures taken.
“Preliminary results show that implementing lockdown measures when infections are in a full exponential growth phase poses serious limitations for their success,” said Faranda.
###
For more information:
Larry Frum
media@aip.org
301-209-3090
Article Title
Authors
Davide Faranda, Isaac Pérez Castillo, Oliver Hulme, Aglaé Jezequel, Jeroen Lamb, Yuruzu Sato, and Erica L. Thompson
Author Affiliations
CNRS